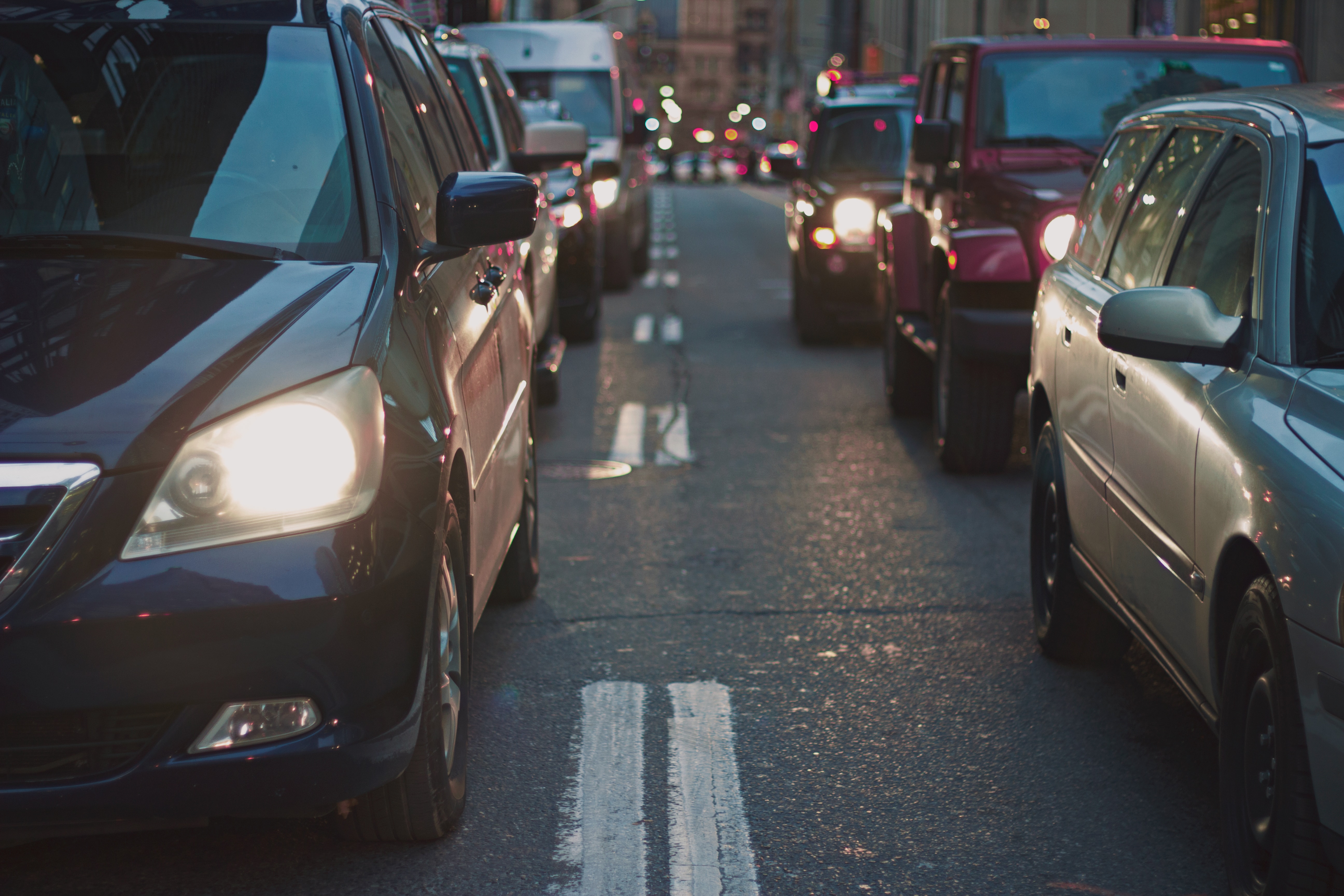
End of Traffic Jams? SDU Professor Develops AI-Controlled Traffic Signal to Reduce CO2 Emissions, Noise, and Fuel Consumption
Today, traffic lights are typically set to change at fixed time intervals, but this can be significantly improved with artificial intelligence that can continuously predict traffic developments and adjust the lights accordingly. Professor Sadok Ben Yahia from the University of Southern Denmark has developed a new system that yields remarkably positive results in a simulated test.
Traffic jams are not just annoying; they also have negative impacts on the economy, public health, road safety, the environment, and the climate. Therefore, there is a good reason to work on new intelligent systems to control traffic lights, ensuring smoother traffic flow in cities and minimizing traffic congestion.
This is precisely what Professor of Data Science Sadok Ben Yahia from the University of Southern Denmark has done. He has developed an AI system called EcoLight+ that can continuously predict traffic behavior and adjust traffic lights accordingly. In a simulated test over three months at one of the busiest intersections in Tallinn, Estonia, Sadok Ben Yahia's system demonstrated significant reductions in CO2 emissions, noise, and estimated fuel consumption.
As reported in the journal "Knowledge and Information Systems," EcoLight+ achieved a noise reduction of 74.6%, reduced CO2 emissions by 63.78%, and decreased fuel consumption by 69.61% compared to conventional time-based traffic lights. It also outperformed other intelligent traffic management models.
"The results are remarkable. They clearly show that our model was the best at finding optimal signal timings throughout the day," says Professor Sadok Ben Yahia. However, he emphasizes the need for further real-world testing after this successful simulated test.
The Negative Impact of Traffic Jams
Traffic jams increase fuel consumption, leading to higher CO2 and particle emissions due to longer engine run times and frequent acceleration and deceleration. Additionally, traffic jams have economic consequences as people waste their time sitting in traffic. In some of the world's most congested cities, like London, according to INRIX, the average driver wastes 156 hours per year, equivalent to nearly four British workweeks. There is also a significant loss of money due to extra fuel consumption.
"We calculated for our test in Tallinn. During the test period, the average gasoline price was $1.9. This means that implementing our system would save $1,689 per hour in fuel costs. That's over $14 million annually," explains Sadok Ben Yahia.
Furthermore, traffic congestion affects road safety, as drivers spend more time on the road, potentially leading to increased stress, frustration, and fatigue, which, in turn, raises the risk of accidents. Additionally, noise pollution is a concern. "I believe we don't focus enough on noise when it comes to traffic jams. We know that traffic noise has various negative effects on our mental and physical health," Sadok Ben Yahia points out.
High-Quality Data
Due to the numerous negative consequences of traffic jams, more and more AI systems have emerged to dynamically manage traffic, surpassing traditional time-based traffic lights. These systems typically receive data from sources such as sensors, cameras on traffic lights, GPS data, and make decisions based on this information.
Professor Sadok Ben Yahia's EcoLight+ system also gathers its data from sensors, cameras, GPS, and even from sensors that measure traffic noise. Subsequently, a process called data fusion takes place.
"When we obtain data from various sources and combine it, we get higher-quality data. This means the model can make more accurate predictions, leading to optimal decision-making for traffic management," Sadok Ben Yahia explains.
"The reason we can achieve such impressive results compared to other systems is related to what is technically known as reinforcement learning. Our model simply functions well."
Moreover, the fact that EcoLight+ relies on measuring noise does not diminish its effectiveness if the vehicle fleet transitions to electric cars. They primarily measure tire noise, Sadok Ben Yahia clarifies.
Currently, the professor is actively seeking partners for the project, raising funding for additional system tests, and planning its implementation at a physical traffic intersection. With the right partners, he anticipates having a prototype ready within six months.